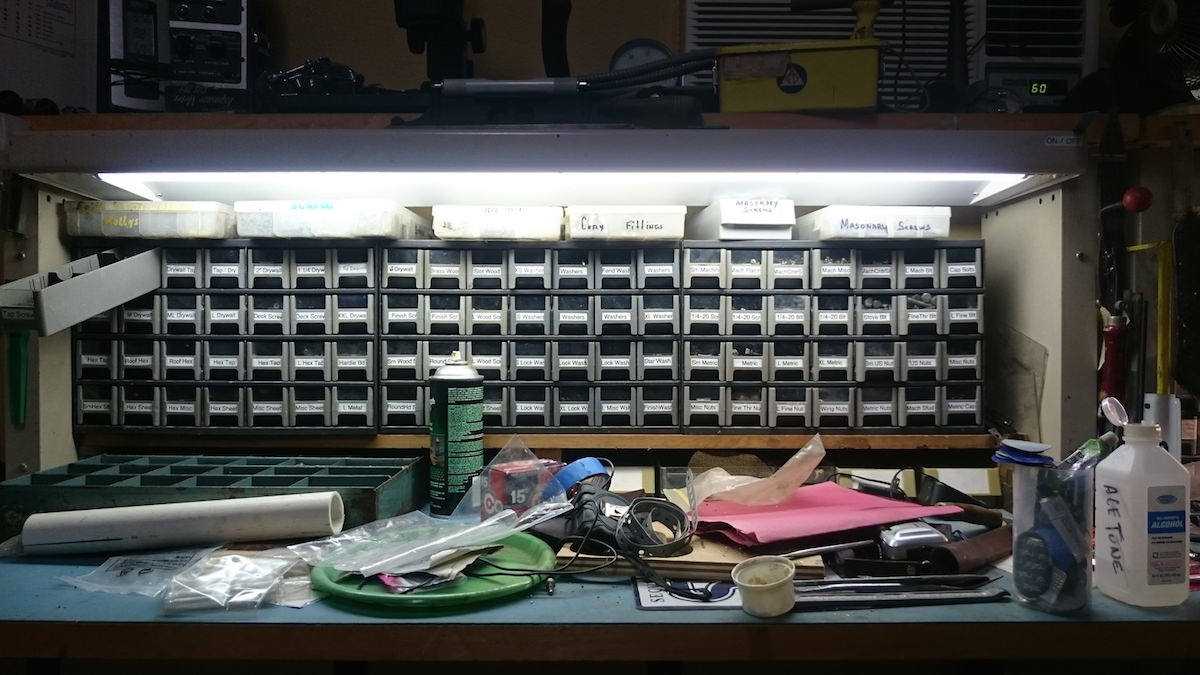
NOTES AND NEWS
How to Read Centile Statistical Vibration Data
For field or building-wide surveys, our practice is to supplement the spatial data gathered across the site with data from (at least) one location gathered over time. This really helps illustrate how much of the observed variability in the spatial data might actually be due to temporal variability. But interpreting these data isn't intuitive for some people.